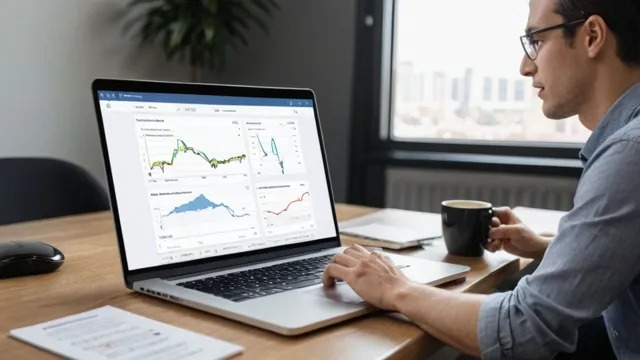
In the dynamic landscape of financial services, the ability to accurately assess creditworthiness is paramount. Traditional credit evaluation methods have long relied on historical data and static metrics, often leading to incomplete assessments and missed opportunities. However, with the advent of advanced machine learning techniques, a new era of credit evaluation has emerged, promising greater accuracy, efficiency, and inclusivity. This paper explores the transformative potential of leveraging ICEBERG scoring, a comprehensive approach to credit assessment, enhanced by advanced machine learning algorithms. By delving into the intricacies of ICEBERG scoring and its integration with cutting-edge technologies, we uncover how financial institutions can unlock untapped financial potential while mitigating risks effectively.
Understanding ICEBERG Scoring :
ICEBERG scoring is a structured approach often used in the fields of risk management, project management, and decision analysis to evaluate and prioritize risks, issues, or projects. The acronym “ICEBERG” stands for different criteria or factors that need to be considered. Here’s a detailed breakdown of how ICEBERG scoring works :
Components of ICEBERG Scoring
Impact : This factor assesses the potential consequences or severity of an issue, risk, or project if it occurs or is implemented. Higher impact typically indicates more significant negative outcomes or benefits.
Cost : This evaluates the financial implications associated with the risk or project. It includes both direct and indirect costs, such as resources, time, and potential lost opportunities.
Exposure : Exposure measures the likelihood or frequency of occurrence. It quantifies how often a risk might materialize or how frequently a project will face critical situations.
Benefit : This criterion assesses the potential positive outcomes or advantages of taking on a project or mitigating a risk. High benefits indicate a more favorable outcome.
Ease of Implementation : This looks at the feasibility and practicality of addressing a risk or executing a project. Factors include available resources, time, technical complexity, and organizational readiness.
Reputation : Reputation considers the potential impact on the organization’s or individual’s reputation. It evaluates how a risk or project might affect stakeholder trust and public perception.
Growth : This factor assesses the potential for future growth or expansion opportunities that a project or risk mitigation might bring. It includes aspects like market potential, scalability, and long-term benefits.
Scoring Methodology
To implement ICEBERG scoring, each factor is assigned a score, typically on a scale from 1 to 10, where 1 represents the lowest impact or relevance, and 10 represents the highest. The process involves several steps:
Define Criteria : Clearly define what each criterion means in the context of your specific application. This ensures consistency and understanding among all stakeholders.
Assess and Score : For each risk, issue, or project, evaluate and score each criterion based on available data, expert judgment, and analysis. This step might involve discussions, workshops, or surveys to gather diverse perspectives.
Weight Criteria (Optional) : Depending on the context, some criteria may be more important than others. Assign weights to each criterion to reflect their relative importance. For instance, impact might be weighted more heavily than ease of implementation.
Calculate Total Scores : Multiply each score by its weight (if weights are used) and sum the results to get a total score for each risk, issue, or project.
Prioritize : Use the total scores to rank the risks, issues, or projects. Higher scores indicate higher priority for action or attention.
Practical Application
ICEBERG scoring can be applied in various contexts, such as :
Risk Management : Identifying and prioritizing risks in a project or organization to focus on the most critical ones.
Project Management : Evaluating potential projects or initiatives to decide which ones to pursue based on their overall scores.
Decision Making : Assessing different options or strategies to determine the best course of action.
Example
Let’s consider an example in project management where a company is evaluating three potential projects. Here’s how ICEBERG scoring might look :
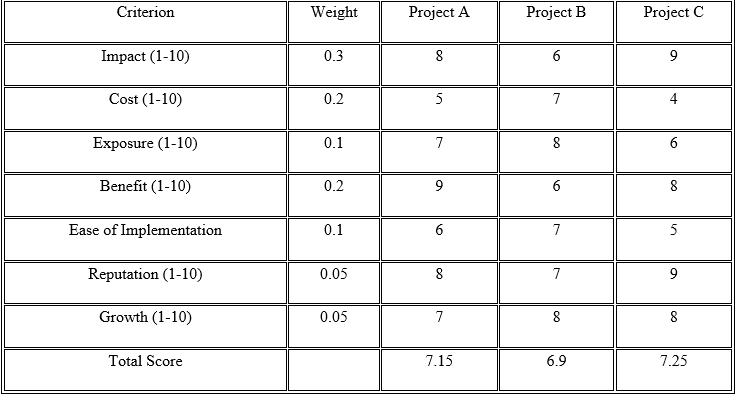
In this example, Project C has the highest total score (7.25), making it the top priority based on the ICEBERG scoring criteria.
Benefits of ICEBERG Scoring
Structured Evaluation : Provides a clear, systematic way to assess and compare different options.
Comprehensive Analysis : Considers multiple dimensions of risk or opportunity, leading to more informed decision-making.
Customizable : Can be tailored to specific contexts by adjusting criteria definitions and weights.
Challenges and Considerations
Subjectivity : Scoring can be subjective, relying on individual judgment. Ensuring a diverse and knowledgeable group of evaluators can mitigate this.
Complexity : The process can be time-consuming, especially if many options need to be evaluated.
Dynamic Factors : Risks and opportunities can change over time, requiring periodic reassessment and updates to scores.
In conclusion, ICEBERG scoring is a versatile and comprehensive tool for evaluating risks, projects, and decisions, providing a structured approach to prioritize actions based on a multi-criteria analysis. By systematically assessing impact, cost, exposure, benefit, ease of implementation, reputation, and growth, organizations can make more informed and strategic decisions.
Challenges with Traditional Approaches :
Traditional approaches in various fields, such as project management, risk management, education, healthcare, and business, often face significant challenges that can impede their effectiveness. Understanding these challenges is crucial for organizations and individuals seeking to improve their practices and outcomes. Here is a detailed exploration of some common challenges associated with traditional approaches across different domains.
1. Lack of Flexibility
Description : Traditional approaches are often rigid and do not adapt well to changes in the environment, technology, or stakeholder needs.
Impact :
Project Management : Fixed plans and methodologies (like Waterfall) struggle with accommodating changes in project scope or requirements, leading to delays and cost overruns.
Education : Static curricula fail to incorporate the latest developments in knowledge and technology, leaving students underprepared for modern challenges.
2. Inefficiency
Description : Traditional methods can be time-consuming and resource-intensive due to their reliance on outdated processes and lack of automation.
Impact :
Healthcare : Manual record-keeping and administrative processes lead to inefficiencies, increasing the workload on healthcare professionals and delaying patient care.
Business : Traditional marketing and operational strategies may not leverage data analytics and digital tools, resulting in missed opportunities and competitive disadvantage.
3. Resistance to Change
Description : Organizations and individuals often resist changing established practices due to comfort with the status quo or fear of the unknown.
Impact :
Organizational Development : Resistance to adopting new technologies or methodologies (like Agile or Lean) can prevent organizations from improving efficiency and innovation.
Education : Teachers and institutions may resist incorporating new teaching methods or technologies, hindering educational reform and student engagement.
4. Siloed Operations
Description : Traditional approaches often encourage siloed thinking, where departments or teams operate independently with little collaboration.
Impact :
Business : Lack of interdepartmental collaboration can lead to inefficiencies, redundancies, and a fragmented customer experience.
Healthcare : Siloed departments may result in poor communication and coordination, negatively impacting patient outcomes and care continuity.
5. Inadequate Risk Management
Description : Traditional risk management practices may not be proactive or comprehensive enough to identify and mitigate risks effectively.
Impact :
Project Management : Failure to anticipate and respond to risks can lead to project failures, budget overruns, and missed deadlines.
Business : Inadequate risk assessment can expose organizations to financial losses, reputational damage, and regulatory penalties.
6. Limited Stakeholder Engagement
Description : Traditional methods often overlook the importance of engaging all stakeholders, leading to decisions that do not fully address their needs and concerns.
Impact :
Public Policy : Policies developed without stakeholder input may face resistance and fail to achieve desired outcomes.
Project Management : Projects that do not consider stakeholder feedback are more likely to encounter opposition and face implementation challenges.
7. Poor Data Utilization
Description : Traditional approaches may not effectively leverage data for decision-making due to lack of infrastructure, skills, or awareness.
Impact :
Business: Companies that do not utilize data analytics may miss insights that could drive strategic decisions and improve performance.
Healthcare: Inadequate data use can result in suboptimal patient care, poor resource allocation, and missed opportunities for improving public health.
8. Inflexible Educational Systems
Description : Traditional educational systems often rely on a one-size-fits-all model, failing to accommodate diverse learning styles and needs.
Impact:
Students : This can lead to disengagement, poor academic performance, and higher dropout rates.
Educators : Teachers may struggle to address the varied needs of their students without flexible curricula and teaching methods.
9. Outdated Technology
Description: Reliance on outdated technology can hamper efficiency and innovation.
Impact :
Business : Companies that do not upgrade their technology infrastructure may fall behind competitors who leverage advanced tools for automation, analytics, and customer engagement.
Healthcare : Use of outdated medical equipment and record-keeping systems can compromise patient care and operational efficiency.
10. Inadequate Training and Development
Description : Traditional approaches often lack continuous training and development opportunities for employees.
Impact :
Workforce : Employees may not acquire the skills needed to adapt to changing job requirements and technological advancements.
Organizational Growth : Lack of investment in human capital can stifle innovation and limit an organization’s ability to compete effectively.
Addressing Traditional Approach Challenges
To overcome the challenges associated with traditional approaches, organizations and individuals can adopt more modern, agile, and data-driven strategies. Here are some steps to consider:
Embrace Flexibility : Adopt flexible methodologies like Agile and Lean to quickly adapt to changes and improve responsiveness.
Leverage Technology : Invest in modern technology and automation to enhance efficiency and reduce manual workloads.
Foster a Culture of Change : Encourage a culture that values innovation and is open to change through continuous training and change management practices.
Promote Collaboration : Break down silos by fostering cross-functional teams and collaborative environments.
Enhance Risk Management : Implement proactive risk management practices that identify and mitigate risks early.
Engage Stakeholders : Actively involve stakeholders in decision-making processes to ensure their needs and concerns are addressed.
Utilize Data Effectively : Build infrastructure and develop skills to leverage data analytics for informed decision-making.
Diversify Educational Approaches : Customize educational methods to accommodate different learning styles and needs.
Update Technology : Regularly update technology to stay current with advancements and maintain competitive advantage.
Invest in Training and Development : Provide continuous learning opportunities to help employees adapt and grow with the organization.
By addressing these challenges, organizations can improve their effectiveness, efficiency, and adaptability in a rapidly changing world.
Challenges with Traditional Approaches :
Traditional approaches in various fields, such as project management, risk management, education, healthcare, and business, often face significant challenges that can impede their effectiveness. Understanding these challenges is crucial for organizations and individuals seeking to improve their practices and outcomes. Here is a detailed exploration of some common challenges associated with traditional approaches across different domains.
1. Lack of Flexibility
Description : Traditional approaches are often rigid and do not adapt well to changes in the environment, technology, or stakeholder needs.
Impact :
Project Management : Fixed plans and methodologies (like Waterfall) struggle with accommodating changes in project scope or requirements, leading to delays and cost overruns.
Education : Static curricula fail to incorporate the latest developments in knowledge and technology, leaving students underprepared for modern challenges.
2. Inefficiency
Description : Traditional methods can be time-consuming and resource-intensive due to their reliance on outdated processes and lack of automation.
Impact :
Healthcare : Manual record-keeping and administrative processes lead to inefficiencies, increasing the workload on healthcare professionals and delaying patient care.
Business : Traditional marketing and operational strategies may not leverage data analytics and digital tools, resulting in missed opportunities and competitive disadvantage.
3. Resistance to Change
Description : Organizations and individuals often resist changing established practices due to comfort with the status quo or fear of the unknown.
Impact :
Organizational Development : Resistance to adopting new technologies or methodologies (like Agile or Lean) can prevent organizations from improving efficiency and innovation.
Education : Teachers and institutions may resist incorporating new teaching methods or technologies, hindering educational reform and student engagement.
4. Siloed Operations
Description : Traditional approaches often encourage siloed thinking, where departments or teams operate independently with little collaboration.
Impact :
Business : Lack of interdepartmental collaboration can lead to inefficiencies, redundancies, and a fragmented customer experience.
Healthcare : Siloed departments may result in poor communication and coordination, negatively impacting patient outcomes and care continuity.
5. Inadequate Risk Management
Description : Traditional risk management practices may not be proactive or comprehensive enough to identify and mitigate risks effectively.
Impact :
Project Management : Failure to anticipate and respond to risks can lead to project failures, budget overruns, and missed deadlines.
Business : Inadequate risk assessment can expose organizations to financial losses, reputational damage, and regulatory penalties.
6. Limited Stakeholder Engagement
Description : Traditional methods often overlook the importance of engaging all stakeholders, leading to decisions that do not fully address their needs and concerns.
Impact :
Public Policy : Policies developed without stakeholder input may face resistance and fail to achieve desired outcomes.
Project Management : Projects that do not consider stakeholder feedback are more likely to encounter opposition and face implementation challenges.
7. Poor Data Utilization
Description : Traditional approaches may not effectively leverage data for decision-making due to lack of infrastructure, skills, or awareness.
Impact :
Business: Companies that do not utilize data analytics may miss insights that could drive strategic decisions and improve performance.
Healthcare: Inadequate data use can result in suboptimal patient care, poor resource allocation, and missed opportunities for improving public health.
8. Inflexible Educational Systems
Description : Traditional educational systems often rely on a one-size-fits-all model, failing to accommodate diverse learning styles and needs.
Impact:
Students : This can lead to disengagement, poor academic performance, and higher dropout rates.
Educators : Teachers may struggle to address the varied needs of their students without flexible curricula and teaching methods.
9. Outdated Technology
Description: Reliance on outdated technology can hamper efficiency and innovation.
Impact :
Business : Companies that do not upgrade their technology infrastructure may fall behind competitors who leverage advanced tools for automation, analytics, and customer engagement.
Healthcare : Use of outdated medical equipment and record-keeping systems can compromise patient care and operational efficiency.
10. Inadequate Training and Development
Description : Traditional approaches often lack continuous training and development opportunities for employees.
Impact :
Workforce : Employees may not acquire the skills needed to adapt to changing job requirements and technological advancements.
Organizational Growth : Lack of investment in human capital can stifle innovation and limit an organization’s ability to compete effectively.
Addressing Traditional Approach Challenges
To overcome the challenges associated with traditional approaches, organizations and individuals can adopt more modern, agile, and data-driven strategies. Here are some steps to consider:
Embrace Flexibility : Adopt flexible methodologies like Agile and Lean to quickly adapt to changes and improve responsiveness.
Leverage Technology : Invest in modern technology and automation to enhance efficiency and reduce manual workloads.
Foster a Culture of Change : Encourage a culture that values innovation and is open to change through continuous training and change management practices.
Promote Collaboration : Break down silos by fostering cross-functional teams and collaborative environments.
Enhance Risk Management : Implement proactive risk management practices that identify and mitigate risks early.
Engage Stakeholders : Actively involve stakeholders in decision-making processes to ensure their needs and concerns are addressed.
Utilize Data Effectively : Build infrastructure and develop skills to leverage data analytics for informed decision-making.
Diversify Educational Approaches : Customize educational methods to accommodate different learning styles and needs.
Update Technology : Regularly update technology to stay current with advancements and maintain competitive advantage.
Invest in Training and Development : Provide continuous learning opportunities to help employees adapt and grow with the organization.
By addressing these challenges, organizations can improve their effectiveness, efficiency, and adaptability in a rapidly changing world.
Harnessing Advanced Machine Learning :
Advanced machine learning techniques have revolutionized various industries, offering unprecedented opportunities for organizations to extract insights, make predictions, automate processes, and drive innovation. Harnessing the power of advanced machine learning requires a deep understanding of its capabilities, limitations, and best practices. In this comprehensive guide, we explore the key concepts, applications, and strategies for leveraging advanced machine learning effectively.
Understanding Advanced Machine Learning
Advanced machine learning refers to sophisticated algorithms and techniques that enable computers to learn from data, identify patterns, and make predictions without being explicitly programmed. Unlike traditional machine learning, which focuses on tasks such as classification and regression, advanced machine learning encompasses a broader range of techniques, including deep learning, reinforcement learning, and unsupervised learning.
Key Techniques in Advanced Machine Learning
Deep Learning : Deep learning is a subset of machine learning that uses neural networks with multiple layers to learn representations of data. It excels at tasks such as image recognition, natural language processing, and speech recognition, where large amounts of data and complex patterns are involved.
Reinforcement Learning : Reinforcement learning is a type of machine learning where an agent learns to make decisions by interacting with an environment and receiving feedback in the form of rewards or penalties. It is well-suited for tasks such as game playing, robotics, and autonomous vehicle control.
Unsupervised Learning : Unsupervised learning involves training algorithms on unlabeled data to discover hidden patterns or structures. Clustering and dimensionality reduction are common techniques used in unsupervised learning, which has applications in anomaly detection, customer segmentation, and data compression.
Generative Adversarial Networks (GANs) : GANs are a class of deep learning models that consist of two neural networks, a generator and a discriminator, trained simultaneously to generate realistic synthetic data. GANs are widely used in image generation, data augmentation, and style transfer.
Applications of Advanced Machine Learning
Image and Video Analysis : Advanced machine learning techniques, such as convolutional neural networks (CNNs), are used for tasks such as object detection, image classification, and video summarization. Applications include medical image analysis, surveillance, and autonomous driving.
Natural Language Processing (NLP) : NLP techniques powered by deep learning, such as recurrent neural networks (RNNs) and transformer models, enable machines to understand and generate human language. Applications include machine translation, sentiment analysis, and chatbots.
Predictive Analytics : Advanced machine learning algorithms are used to analyze historical data and make predictions about future events or outcomes. Applications include financial forecasting, demand prediction, and personalized recommendations.
Healthcare : Machine learning is transforming healthcare by enabling early disease detection, personalized treatment plans, and medical image analysis. Deep learning models are used for tasks such as diagnosing diseases from medical images, predicting patient outcomes, and drug discovery.
Autonomous Systems : Reinforcement learning is used to train autonomous systems, such as robots and self-driving cars, to make decisions in dynamic and uncertain environments. These systems learn to navigate, interact with the environment, and achieve specific goals through trial and error.
Fraud Detection : Machine learning algorithms are used to detect fraudulent activities in various domains, including finance, insurance, and e-commerce. Techniques such as anomaly detection and pattern recognition help identify suspicious behavior and prevent fraudulent transactions.
Best Practices for Harnessing Advanced Machine Learning
Data Quality : High-quality data is essential for training accurate machine learning models. Organizations should invest in data collection, cleaning, and preprocessing to ensure the reliability and relevance of their datasets.
Model Selection : Choosing the right model architecture and algorithms depends on the specific task and dataset. Organizations should experiment with different techniques and evaluate their performance using appropriate metrics.
Feature Engineering : Feature engineering plays a crucial role in model performance. Organizations should carefully select and engineer features that capture relevant information from the data and improve predictive accuracy.
Hyperparameter Tuning : Hyperparameters control the behavior and performance of machine learning models. Organizations should use techniques such as grid search and random search to optimize hyperparameters and improve model performance.
Validation and Testing : Proper validation and testing are essential for assessing the generalization and robustness of machine learning models. Organizations should use techniques such as cross-validation and holdout validation to evaluate models on unseen data.
Interpretability and Transparency : Understanding how machine learning models arrive at their predictions is crucial for trust and accountability. Organizations should use techniques such as feature importance analysis and model explainability methods to interpret and explain model decisions.
Ethical Considerations : Machine learning models can perpetuate biases present in the data and have unintended consequences. Organizations should consider ethical implications and fairness principles when designing, training, and deploying machine learning systems.
Continuous Monitoring and Maintenance : Machine learning models degrade over time as data distributions shift and environments change. Organizations should monitor model performance regularly and retrain models periodically to ensure they remain accurate and up-to-date.
Case Studies of Successful Implementations
AlphaGo : DeepMind’s AlphaGo used deep reinforcement learning to defeat world champion Go players, demonstrating the power of advanced machine learning in complex decision-making tasks.
Google Translate : Google Translate employs deep learning techniques to provide accurate and fluent translations between different languages, enabling seamless communication across cultures.
Amazon Recommendation System : Amazon’s recommendation system uses machine learning algorithms to analyze customer behavior and preferences, providing personalized product recommendations that drive sales and customer satisfaction.
Tesla Autopilot : Tesla’s Autopilot system uses deep learning and reinforcement learning to enable autonomous driving capabilities, enhancing vehicle safety and convenience.
The integration of ICEBERG scoring with advanced machine learning techniques represents a powerful synergy between structured decision-making frameworks and cutting-edge predictive analytics. By leveraging the strengths of both approaches, organizations can enhance their ability to assess and prioritize risks, projects, and decisions in a more dynamic and data-driven manner. Here’s an in-depth exploration of how ICEBERG scoring can be integrated with advanced machine learning :
1. Data-Driven Risk Assessment
Traditional ICEBERG scoring relies on expert judgment and historical data for assessing risks and opportunities. By integrating advanced machine learning algorithms, organizations can augment this process by analyzing large datasets to identify patterns, trends, and correlations that may not be immediately apparent to human evaluators. Machine learning models can process structured and unstructured data sources to provide more accurate risk assessments and predictions.
2. Predictive Analytics for Impact and Exposure
Machine learning algorithms, such as regression analysis, decision trees, and neural networks, can be trained on historical data to predict the potential impact and exposure of risks or projects. These models can consider a wide range of variables and factors to forecast outcomes more accurately than traditional scoring methods. For example, in project management, predictive models can analyze project parameters, stakeholder dynamics, market conditions, and external factors to anticipate project risks and their potential impact on cost, schedule, and quality.
3. Real-Time Monitoring and Adaptive Scoring
Machine learning algorithms excel at processing streaming data and detecting anomalies in real-time. Organizations can leverage this capability to continuously monitor risks and projects and adjust ICEBERG scores dynamically based on changing conditions. For instance, in financial risk management, machine learning models can analyze market data and news feeds to identify emerging risks and update risk scores accordingly, enabling proactive risk mitigation strategies.
4. Personalized Weighting and Prioritization
Machine learning algorithms can analyze historical decision-making data to learn from past outcomes and preferences, allowing organizations to personalize weighting and prioritization criteria based on their specific goals and objectives. By incorporating feedback loops and reinforcement learning techniques, organizations can optimize ICEBERG scoring models over time to reflect evolving priorities and preferences.
5. Natural Language Processing for Reputation Analysis
Reputation is a critical factor in ICEBERG scoring, especially in industries where trust and public perception play a significant role. Natural Language Processing (NLP) techniques can analyze textual data from social media, news articles, and online reviews to assess an organization’s reputation and sentiment. Sentiment analysis algorithms can categorize text as positive, negative, or neutral, providing valuable insights into public opinion and potential reputational risks.
6. Ensemble Models for Robust Decision Making
Ensemble learning techniques, such as random forests and gradient boosting, can combine multiple machine learning models to improve predictive accuracy and robustness. Organizations can build ensemble models that integrate ICEBERG scoring with various machine learning algorithms to leverage the strengths of each approach. By aggregating predictions from diverse models, organizations can make more reliable and robust decisions while mitigating the risk of overfitting or bias.
7. Explainable AI for Transparent Decision Making
As organizations rely more on advanced machine learning techniques, the need for transparent and explainable AI becomes paramount. Techniques such as LIME (Local Interpretable Model-agnostic Explanations) and SHAP (SHapley Additive exPlanations) can provide insights into how machine learning models arrive at their predictions. By enhancing the interpretability of ICEBERG scoring models, organizations can gain stakeholders’ trust and confidence in the decision-making process.
8. Continuous Learning and Adaptation
Machine learning models can learn and adapt from new data, allowing ICEBERG scoring systems to evolve and improve over time. Organizations can implement feedback mechanisms to incorporate new information and user feedback into the scoring process, enabling continuous learning and adaptation. By embracing a culture of experimentation and iteration, organizations can refine ICEBERG scoring models to better reflect the complexities of their operating environment and stakeholders’ needs.
Benefits of Integrating ICEBERG Scoring with Advanced Machine Learning
Enhanced Predictive Accuracy: Machine learning algorithms can provide more accurate risk assessments and predictions, enabling organizations to make more informed decisions .
Real-Time Monitoring and Adaptation : Organizations can react quickly to changing conditions by leveraging real-time data analysis and dynamic scoring mechanisms.
Personalized Decision Making : Machine learning models can tailor scoring criteria and weights to individual preferences and objectives, improving the relevance and effectiveness of decision-making processes.
Transparent and Explainable Decisions : By incorporating explainable AI techniques, organizations can enhance the transparency and interpretability of ICEBERG scoring models, fostering trust and buy-in from stakeholders.
Continuous Improvement : Machine learning enables ICEBERG scoring systems to evolve and improve over time through continuous learning and adaptation, ensuring they remain relevant and effective in a dynamic environment.
Challenges and Considerations
Data Quality and Availability: Integrating machine learning requires access to high-quality, relevant data, which may not always be readily available or easily accessible.
Model Interpretability : As machine learning models become more complex, ensuring their interpretability and transparency becomes challenging, particularly in highly regulated industries.
Bias and Fairness : Machine learning models may inadvertently perpetuate biases present in the data, leading to unfair or discriminatory outcomes if not properly addressed and mitigated.
Resource Requirements : Building and maintaining machine learning models requires significant computational resources, expertise, and investment, which may be prohibitive for some organizations.
Case Study : Implementing ICEBERG Scoring with Advanced Machine Learning
Company Background
ABC Corporation is a multinational conglomerate with diverse business interests, including manufacturing, retail, and technology services. With a global footprint and a complex operating environment, ABC Corporation faces numerous risks and opportunities across its business units.
Challenge
ABC Corporation recognized the need to improve its risk management processes and decision-making capabilities to stay competitive in a rapidly evolving market. The company sought to implement a more robust and data-driven approach to assess and prioritize risks, projects, and investments across its diverse portfolio.
Solution
ABC Corporation decided to implement ICEBERG scoring integrated with advanced machine learning techniques to enhance its risk management practices. The following steps outline how the company implemented this solution :
1. Data Collection and Preparation
ABC Corporation gathered historical data on past projects, investments, and risk incidents from various sources, including internal databases, financial records, and project reports. The data encompassed a wide range of variables, including project parameters, financial metrics, market conditions, and stakeholder feedback. The data were cleaned, standardized, and prepared for analysis to ensure accuracy and consistency.
2. Feature Engineering and Model Development
The data were used to develop machine learning models to predict the impact, exposure, and likelihood of future risks and projects. ABC Corporation employed a team of data scientists and risk management experts to perform feature engineering, selecting relevant variables and creating new features to improve predictive accuracy. Various machine learning algorithms, such as regression analysis, decision trees, and neural networks, were tested and evaluated to identify the most effective models for each use case.
3. Model Training and Validation
The machine learning models were trained on historical data using techniques such as cross-validation and hyperparameter tuning to optimize performance. ABC Corporation validated the models using holdout datasets and out-of-sample testing to ensure their robustness and generalizability. The models were refined iteratively based on feedback from domain experts and stakeholders to improve their accuracy and reliability.
4. Integration with ICEBERG Scoring Framework
The machine learning models’ predictions were integrated with the ICEBERG scoring framework to assess and prioritize risks, projects, and investments. Each criterion in the ICEBERG framework was informed by the predictions generated by the machine learning models, providing a more accurate and data-driven assessment of each risk or opportunity’s impact, exposure, and likelihood. Stakeholders were engaged throughout the process to provide input and validate the scoring criteria.
5. Real-Time Monitoring and Adaptation
ABC Corporation implemented a real-time monitoring system to track changes in risk factors, market conditions, and other relevant variables. The machine learning models were deployed in a cloud-based environment capable of processing streaming data and updating risk scores dynamically. Automated alerts and notifications were generated to notify decision-makers of significant changes or emerging risks, enabling proactive risk mitigation strategies.
6. Continuous Learning and Improvement
ABC Corporation established a feedback loop to collect data on the outcomes of risk management decisions and project implementations. This data were used to update and improve the machine learning models continuously. By incorporating feedback from real-world experiences, ABC Corporation iteratively refined its risk management processes and decision-making frameworks to better align with its strategic objectives and stakeholders’ needs.
Results
By implementing ICEBERG scoring integrated with advanced machine learning techniques, ABC Corporation achieved the following outcomes:
Improved Decision Making: The integration of machine learning enhanced the accuracy and reliability of risk assessments and project evaluations, enabling ABC Corporation to make more informed and data-driven decisions.
Proactive Risk Management : The real-time monitoring capabilities allowed ABC Corporation to identify and mitigate risks more effectively, reducing the likelihood of costly incidents and disruptions.
Optimized Resource Allocation : By prioritizing risks and projects based on their predicted impact and likelihood, ABC Corporation optimized resource allocation and investment decisions, maximizing returns and minimizing losses.
Enhanced Stakeholder Engagement : The transparent and data-driven approach to risk management fostered trust and collaboration among stakeholders, leading to more effective risk mitigation strategies and project implementations.
Continuous Improvement : The feedback loop enabled ABC Corporation to continuously learn and adapt its risk management processes, ensuring they remained relevant and effective in a dynamic and evolving business environment.
Conclusion :
Unlocking financial potential through ICEBERG scoring with advanced machine learning represents a paradigm shift in credit evaluation. By embracing a holistic approach that considers diverse dimensions of an individual’s financial profile, financial institutions can make more informed decisions, mitigate risks effectively, and foster greater financial inclusivity. As technology continues to evolve, the marriage of ICEBERG scoring and advanced machine learning holds immense promise for reshaping the future of financial services and empowering individuals to achieve their financial goals.
I am genuinely amazed by your profound understanding and excellent writing style. Your depth of knowledge is evident in every sentence. It’s evident that you invest a great deal of effort into delving into your topics, and the results pays off. Thank you for sharing this valuable knowledge. Keep on enlightening us! https://rochellemaize.com
I am genuinely amazed with your profound understanding and stellar way of expressing complex ideas. Your expertise clearly stands out in every sentence. It’s clear that you spend considerable time into researching your topics, and this effort pays off. Thank you for sharing such detailed information. Continue the excellent job! https://www.elevenviral.com
Thanks for the points shared on your blog. Another thing I would like to say is that weight reduction is not supposed to be about going on a fad diet and trying to lose as much weight as you’re able in a set period of time. The most effective way to shed weight is by consuming it slowly and using some basic suggestions which can assist you to make the most through your attempt to lose weight. You may be aware and already be following many of these tips, however reinforcing know-how never affects.
Howdy, i read your blog occasionally and i
own a similar one and i was just wondering if you
get a lot of spam comments? If so how do you protect
against it, any plugin or anything you can suggest?
I get so much lately it’s driving me insane so any assistance is very much appreciated.
Your means of describing everything in this paragraph is truly good, all be capable of easily understand it,
Thanks a lot.
I’m thoroughly captivated by the deep insights and excellent ability to convey information. Your depth of knowledge clearly stands out in every sentence. It’s evident that you invest a great deal of effort into researching your topics, and that effort pays off. We appreciate your efforts in sharing this valuable knowledge. Continue the excellent job! https://www.elevenviral.com
That is a very good tip especially to those new to the
blogosphere. Brief but very precise info… Appreciate your sharing this one.
A must read post!
Keep this going please, great job!
I simply desired to say thanks all over again. I am not sure the things I would’ve sorted out without the entire recommendations discussed by you directly on this topic. Completely was the challenging dilemma in my circumstances, but taking a look at your well-written style you treated that forced me to weep with fulfillment. Now i’m thankful for your guidance as well as hope you comprehend what a powerful job that you are undertaking training people today via a web site. I’m certain you haven’t come across any of us.
Everyone loves what you guys are usually up too. This sort of clever work and exposure! Keep up the amazing works guys I’ve included you guys to my personal blogroll.
There is noticeably a bundle to know about this. I assume you made sure good points in options also.
Today, while I was at work, my cousin stole my iPad and tested to see if it can survive a 40 foot drop, just so she can be a youtube sensation. My iPad is now destroyed and she has 83 views. I know this is entirely off topic but I had to share it with someone!
I’ve learned some important things as a result of your post. I would also like to state that there is a situation where you will obtain a loan and do not need a cosigner such as a Fed Student Support Loan. However, if you are getting a borrowing arrangement through a conventional loan company then you need to be made ready to have a co-signer ready to assist you. The lenders may base that decision using a few issues but the most important will be your credit ratings. There are some lenders that will in addition look at your work history and decide based on that but in many instances it will hinge on your rating.
Its like you read my mind! You appear to know so much about this, like you wrote the book in it or something. I think that you can do with a few pics to drive the message home a bit, but instead of that, this is excellent blog. A fantastic read. I’ll definitely be back.
Hello there, I found your web site via Google while searching for a related topic, your site came up, it looks great. I’ve bookmarked it in my google bookmarks.
I have read several good stuff here. Certainly value bookmarking for revisiting. I wonder how much effort you set to make this sort of excellent informative web site.