Fraudulent activities in the financial sector continue to rise, costing businesses billions annually. Among the most targeted areas is bank statement analysis, a critical process used by financial institutions to verify the legitimacy of transactions and detect anomalies. As fraudsters become more sophisticated, leveraging technology and innovative schemes, it’s imperative to adopt advanced techniques to safeguard assets and customer trust. Let’s explore how financial institutions can combat fraud effectively through bank statement analysis.
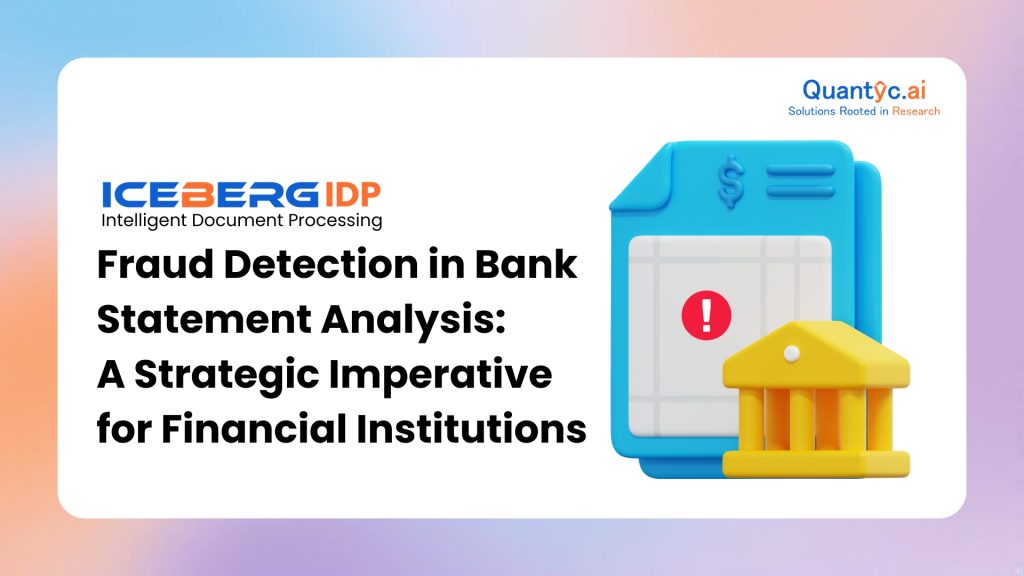
Understanding Fraud in Bank Statements
Fraud in bank statements often involves manipulating financial records to present a false reality. This can include fabricating transactions, overstating income, or concealing liabilities. Fraudulent bank statements are not just used by individuals attempting to secure loans or credit but also by organizations involved in more elaborate schemes.
Common Types of Bank Statement Fraud:
- Fictitious Transactions: Recording fake deposits or expenditures.
- Income Overstatement: Inflating income figures to secure loans or financing.
- Omission of Liabilities: Hiding existing debts to improve creditworthiness.
- Identity Fraud: Using stolen or fabricated identities to create fraudulent accounts.
Such activities compromise institutional integrity and can result in legal and reputational repercussions.
Techniques for Detecting Fraud in Bank Statements
Financial institutions are employing a range of strategies, combining traditional forensic practices with cutting-edge technologies. Below are some key methodologies:
1. Advanced Analytics and AI Integration
Artificial Intelligence (AI) and machine learning (ML) are revolutionizing fraud detection. By analyzing vast datasets, these technologies can identify unusual patterns or behaviors indicative of fraud.
- Predictive Analytics: Predictive models analyze historical transaction data to flag high-risk activities.
- Real-Time Monitoring: AI systems can monitor transactions in real-time, identifying red flags such as rapid fund movements or inconsistent account behaviors.
2. Behavioral Analysis
Analyzing the behavioral patterns of account holders can be a game-changer. Unusual spending habits, inconsistent deposit frequencies, or sudden spikes in transaction volume often signal fraudulent activity. Techniques like Peer Group Analysis compare an account’s behavior with similar profiles to detect outliers.
3. Anomaly Detection Models
Anomaly detection algorithms like Isolation Forests and One-Class SVMs (Support Vector Machines) are designed to spot data points that deviate significantly from normal trends. These methods are highly effective for identifying:
- Repeated small-value transactions aimed at evading detection limits.
- Unusual withdrawal or transfer patterns.
4. Forensic Accounting
Traditional forensic accounting remains a cornerstone of fraud detection. By meticulously reviewing financial records and reconciling discrepancies, forensic experts can uncover irregularities that automated systems might miss.
5. Document Verification
Using Intelligent Document Processing (IDP), institutions can validate the authenticity of uploaded bank statements. Advanced IDP systems check for signs of tampering, such as altered fonts, mismatched formatting, or manipulated PDF metadata.
Challenges in Fraud Detection
While the methodologies above enhance fraud detection capabilities, several challenges persist:
- Evolving Tactics: Fraudsters continuously adapt, developing schemes that evade even sophisticated systems.
- Data Overload: Analyzing massive volumes of transactions can strain systems and resources.
- Balancing Accuracy with Customer Experience: Overzealous scrutiny can lead to false positives, negatively impacting legitimate customers.
Emerging Trends in Fraud Detection
As fraud becomes more complex, emerging trends in technology are reshaping how institutions tackle this issue:
- Network-Based Fraud Detection: Algorithms like Personalized PageRank analyze the relationships between accounts to uncover social dynamics indicative of collusion.
- Explainable AI (XAI): Ensuring transparency in AI decisions helps institutions understand why a transaction is flagged as fraudulent, fostering better trust and compliance.
- Blockchain Verification: Blockchain technology is being explored for its ability to create immutable transaction records, reducing the risk of tampering.
The Future of Fraud Detection
Fraud detection is no longer optional—it’s a strategic imperative. Financial institutions must combine technology with human expertise to stay ahead of fraudsters. By adopting a proactive approach that leverages machine learning, behavioral analysis, and forensic tools, organizations can not only mitigate losses but also reinforce customer confidence.
Learn more about how IDP systems validate documents.
Sources
- Effectiv AI – Predictive Analytics for Fraud Detection
- Wikipedia – Data Analysis for Fraud Detection
- Arya.ai – Fraud Detection in Banking
- Arxiv.org – Personalized PageRank for Fraud Detection
- The Australian – AI in Financial Crime
- Wikipedia – Forensic Accounting