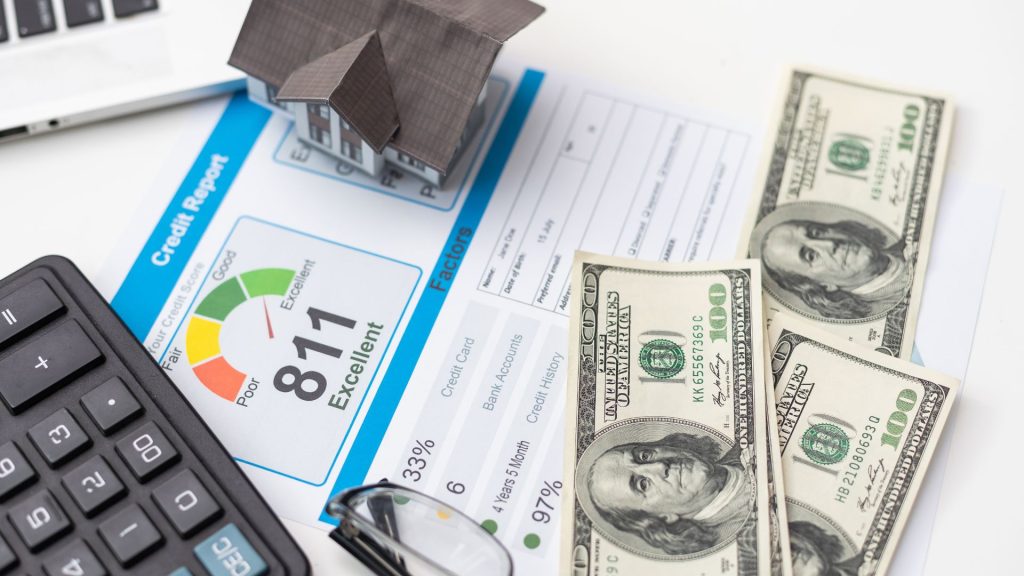
Estimating the creditworthiness of a borrower through credit scoring is a well-established and evolved process in financial institutions. This is as it should be, given the importance of assessing potential defaulters correctly in advance for mitigating lending risk. For some time now, lending organizations have relied on credit scoring software for helping them in credit decisions.
Software-aided credit approval solutions can be either an organization-specific system tailor-made for its requirements, or a score obtained from an external credit rating agency.
Credit scores from an external agency come with both strengths and weaknesses.
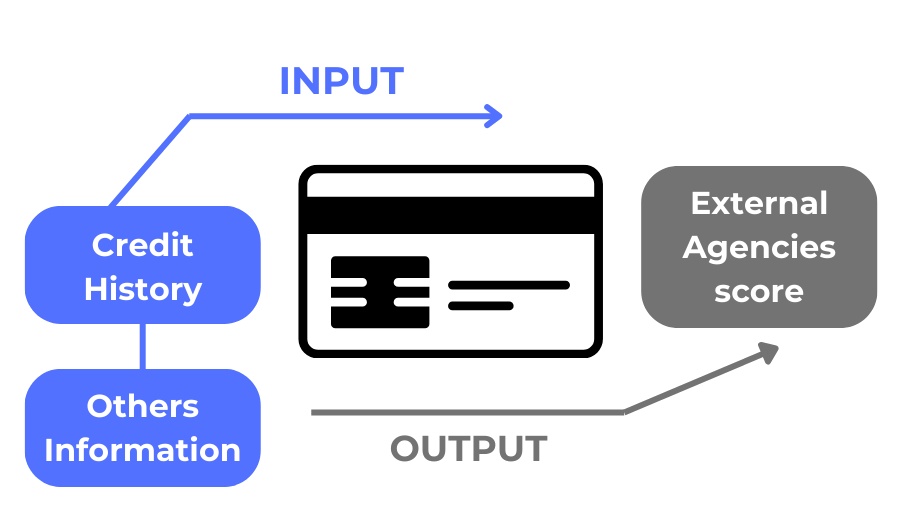
A lending organization can request an external credit rating agency for the credit score of a potential borrower by paying a fee. Credit bureau scoring solutions use sophisticated models and have the benefit of a very large database to analyse and create scoring models.
On the other hand, the scoring by external rating agencies suffers from a few limitations too, aside from appearing as a black box to the financial institution (FI) that uses it.
Firstly, the data they use might not be representative of the customer profile to which a given FI is offering loans, nor be tailored to its specific loan products. The database of the rating agency might comprise mostly of credit card payment data, while the company might be using the rating for disbursing mortgage loan, just to take an example.
In-house assessment solutions address some of the inherent limitations of credit bureau scoring systems.
In an increasing trend, contemporary financial institutions are acquiring credit scoring solutions tailor-made for themselves. The solutions factor the customer demographics specific to their business, and the kind of loan products they normally disburse. It is not difficult to see that such solutions are likely to be more relevant or aligned to their particular nature of business, potentially resulting in more reliable credit scores for their purpose.
All lending organizations have always had internal policies and well-defined in-house rules for evaluating the creditworthiness of their potential borrower’s years before software solutions started being made. The earlier software implementations of credit scoring systems simply transposed the existing manually performed rules into software, with varying degrees of hard-coding or parameterization.
Such systems, generally called Rule-based solutions, define an organization’s credit approval policies, guidelines as well as accumulated business knowledge in the area, usually using a powerful interactive rule engine.
Quite recently though, a new kind of programming technology – in fact, a new way of thinking about solutions, has taken the world by storm. This is Artificial Intelligence and its special case, Machine Learning. The latest advancements in this new and exciting field now enable us to foresee if a borrower is likely to repay the loan in a measurable way using an entirely new way of evaluation.
Credit Scoring solutions powered by Artificial intelligence run on the organization’s own historical data of previously disbursed and closed loans, including both ‘good’ and non-performing loans. Solutions built using machine learning models give an accurate prediction of the probability of loan default. What gives them the edge is their internal sophistication and relevance, because models are fine-tuned on the organization’s own data.
The other big plus comes from the fact that models can be designed to automatically improve in accuracy over time as more real recent data become available. Whether rule-based on AI-based, in-house credit scoring solutions bring a few benefits to the table for the organization. The institution can potentially build different models for different kinds of loans offered by them. For example, it is logical that the evaluation criteria for mortgages are different from those used for student or automobile loans.
In-house solutions are in your control and need not cost you every time you ask for a credit evaluation. Therefore, you can run a credit assessment of all your running loans regularly during the loan life-cycle. This reduces the risk of a sudden drop in credit worthiness of some of the borrowers since the loan was disbursed. This advance warning allows some proactive action to mitigate loan default risk.
While implementing the in-house software, the organization can ensure that the credit scoring component supplies a compatible API and is seamlessly interfaced with the financial institution’s existing loan origination or approval system, providing a smooth unbroken workflow to the users, something not guaranteed for an external solution
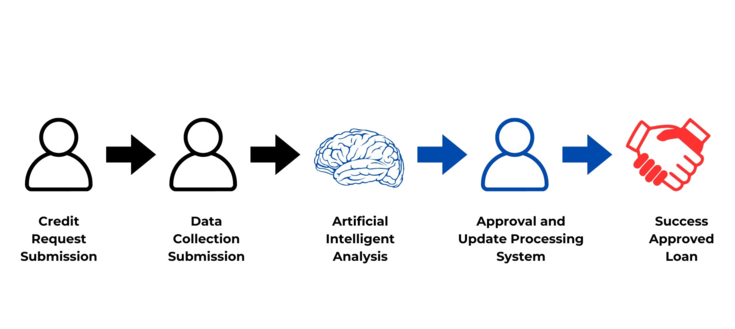
Choosing the right credit scoring system does not have to be a stressful task.
So, which kind of credit scoring solution should a financial institution choose? In-house solutions are clearly appealing and bring many advantages to the table. Should one go for them, Leave the long-standing industry-wide acceptability of credit scores from a reputed rating agency?
Even amongst in-house options, the solutions powered by AI are obviously very attractive, but should financial institutions forgot the easy, comforting familiarity of the existing rules and policies that have served them for so long?
The good news is that it is not necessary to settle the debate on whether a credit score from a bureau will work better, or that from in-house solutions. You could use all these scores, and generate a blended score in some defined proportion. As time goes, and results are seen, adjust or fine-tune the relative weights of the different methods till the optimum balance is achieved.
A smart, modern credit evaluation solution will clearly bring a few standout attributes to the table.
Top-tier credit scoring solutions will spare you the stress and risk of choosing a scoring method, either in-house or from the rating agency, without data-backed evidence either way. It should allow users to define policy-based rules, and also generate a machine trained model using historical loan data. Nor should it prevent the organization from obtaining scores from an external rating agency as well. Credit scoring solution should be able to blend all three scores approach to credit assessment, incorporating rule-based, AI-powered, and external rating agency scores to ensure robustness and accuracy. It should empower users to define policy-based rules and generate machine-trained models using historical loan data, while also allowing integration with external rating agencies.
Moreover, a lending organization might design some products only for a defined class of customer demography, be it women, students or farmers, to take some examples. In some other kinds of loans, the borrower’s age or residency might make her ineligible. The smarter solutions come with a rule engine for defining a set of product-specific eligibility criteria to filter the borrowers for each loan product as per your policy. So, the credit scoring itself may be skipped for the ineligible borrowers.
Going beyond the core function of credit scoring itself, an industrial strength solution should add value through additional features and attributes, the system should provide a rule engine for designing product-specific eligibility criteria, enabling lenders to filter borrowers efficiently and skip credit scoring for ineligible applicants. This solution should feature user-friendly interfaces, eliminating the need for technical expertise, and offer flexibility in data types and input formats. At the end, it should support both standalone and API-driven usage for batched or online processes, catering to diverse workflow needs seamlessly.
An industrial-strength solution should enhance value through insightful visualization of results, offering transparency in algorithm selection, model accuracy, and variable influence. It should facilitate role-based access management, enabling teams to create custom rules or models for different loan products while ensuring data segregation. Moreover, the system should have a low upfront cost, preferably offered through a cloud-based subscription model, with scalability to accommodate business growth efficiently. By meeting these criteria, such a solution not only optimizes credit assessment processes but also enhances decision-making transparency and operational efficiency for lending institutions.
ICEBERG Scoring, the smart and modern AI powered Credit Scoring solution from Quantyc.ai, ticks all these boxes, ready for you to take advantage of.